DeepMind has released a new self-improving AI-powered robot “RoboCat”, which is capable of creating its own training data and teaches itself without any human intervention.
Robots are getting closer to human life, becoming part of households by performing tasks assigned to them & trying to be a friend with humans. But, they’re often only programmed to perform specific new tasks well, which compels for the need of regular programming update to make the robot better by doing new tasks.
Addressing this sigh of constant teaching to robots, a new model by DeepMind will allow robots to self-learn or self-improve itself, avoiding the need of human mediation.
DeepMind, an UK based AI research firm, acquired by Google in 2014, introduced the robot that can self-improve itself, named as “RoboCat”. Earlier DeepMind had released an AI model – Plato, that can self-learn by watching videos and now the technology is coming to robotics.
RoboCat – Self-improving AI Robot
RoboCat is a self-improving AI-powered robotic agent that learns to perform a variety of tasks across different arms and then self-generates new training data to improve its technique.
RoboCat aims to be a master of general-purpose robots, that which can automate a variety of applications and simultaneously learn from its own experiences. Normally general-purpose robots are quite difficult to design or time-consuming, as they require real-world training data for its upgrade of actions. However, RoboCat might help eradicate the hurdle, fitting better for industrial applications.
“RoboCat is the first agent to solve and adapt to multiple tasks and do so across different, real robots,” writes DeepMind.
The robot can pick up a new task with as few as 100 demonstrations, which might help accelerating robotics research, as it reduces the need for human-supervised training.
DeepMind powers RoboCat with its multimodal model “Gato” (Spanish for “cat”), which can process language, images and actions in both simulated and physical environments. “We combined Gato’s architecture with a large training dataset of sequences of images and actions of various robot arms solving hundreds of different tasks.”
How RoboCat self-improves?

After training the robot with an initial dataset, DeepMind launched RoboCat into a “self-improvement” training cycle with a set of previously unseen tasks. It involves five steps for the robot to make it self-learn, which goes as this:
- Collect 100 – 1000 demonstrations of a new task or robot, using a robotic arm controlled by a human.
- Fine-tune RoboCat on this new task/arm, creating a specialised spin-off agent.
- The spin-off agent practises on this new task/arm an average of 10,000 times generating more training data.
- Incorporate the demonstration data and self-generated data into RoboCat’s existing training dataset.
- Train a new version of RoboCat on the new training dataset.
Final result is a robot that is training on a dataset of millions of trajectories, from both real and simulated robotic arms, including self-generated data.
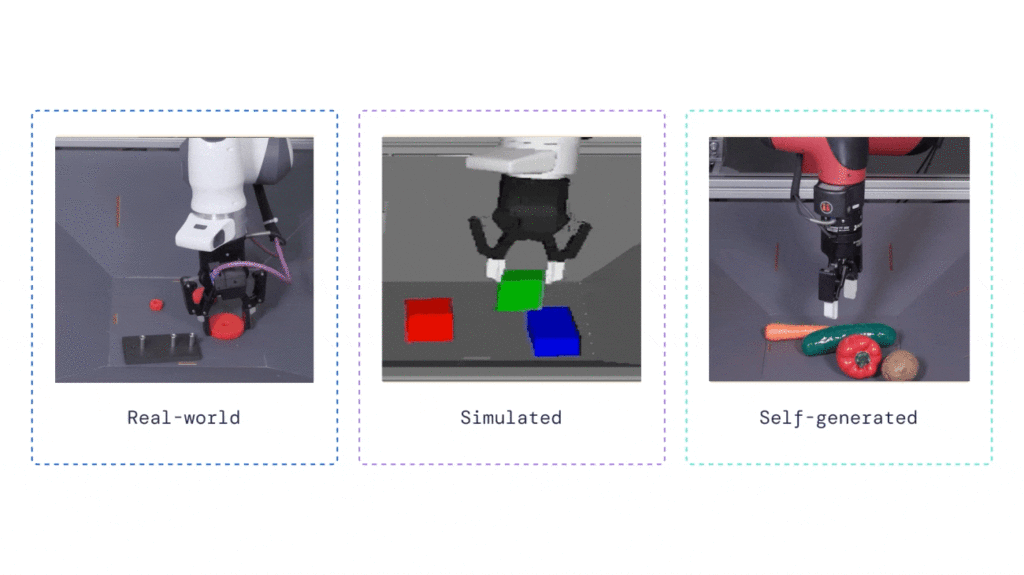
RoboCat excels in learning
RoboCat was also able to adhere to existing physical conditions though it had been trained on different condition. For example, it had been trained on arms with two-pronged grippers, and it was able to adapt to a more complex arm with a three-fingered gripper and twice as many controllable inputs.
RoboCat’s AI model entitles the robot to learn performing tasks by simply watching the demonstrations. After observing 1000 human-controlled demonstrations, collected in just hours, RoboCat could direct this new arm dexterously enough to pick up gears successfully 86% of the time. With the same level of demonstrations, it could adapt to solve tasks that combined precision and understanding, such as removing the correct fruit from a bowl and solving a shape-matching puzzle, which are necessary for more complex control.
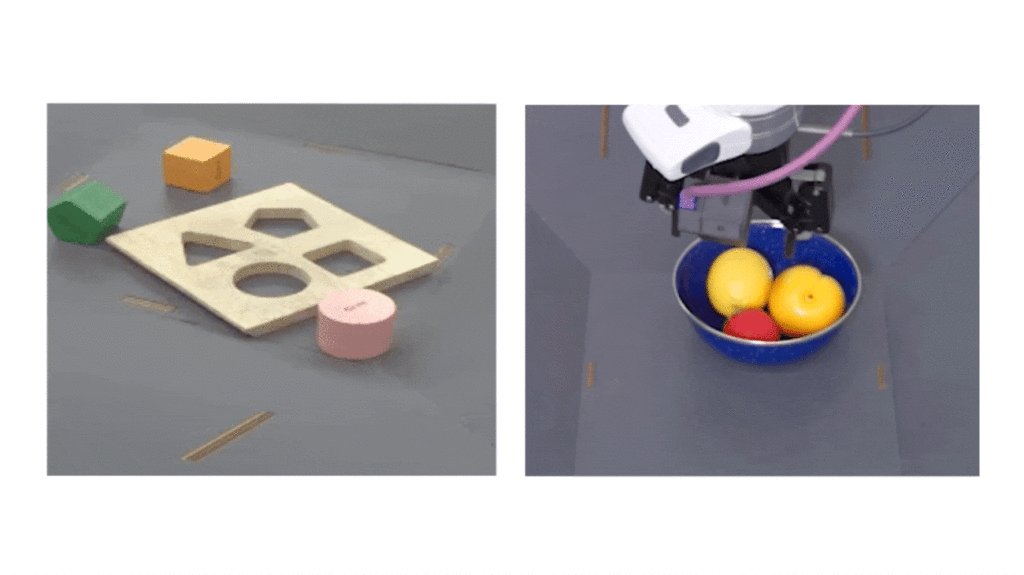
While the initial version of RoboCat was successful only 36% of the time on performing new unseen tasks, the final version showed twice the success-rate – 74% on same tasks.
RoboCat’s training reveals that the more new tasks it learns, the better it gets at learning additional new tasks, likely as any human would widen his/her skills by deepening their learning.
“RoboCat’s ability to independently learn skills and rapidly self-improve, especially when applied to different robotic devices, will help pave the way toward a new generation of more helpful, general-purpose robotic agents,” concludes the paper.
Hope you find the page useful!
(For more such interesting informational, technology and innovation stuffs, keep reading The Inner Detail).
Kindly add ‘The Inner Detail’ to your Google News Feed by following us!